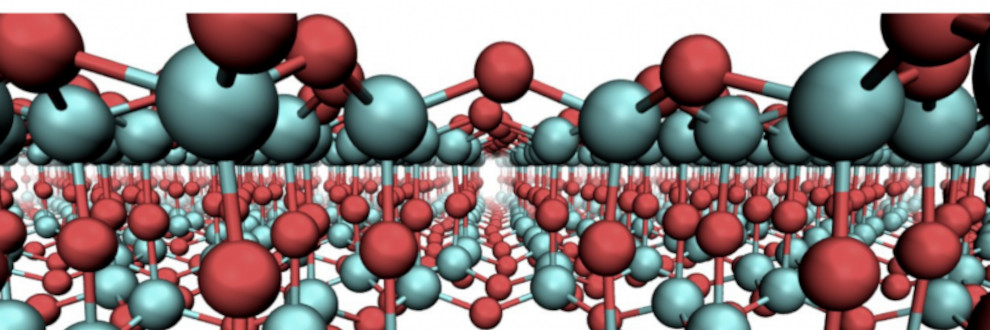
Predicting fracture with artificial intelligence
Being able to predict when and where a material will fracture is a key issue with important industrial implications in the area of device and component monitoring. Researchers from the Center for Complexity and Biosystems and the "Aldo Pontremoli" Department of Physics at the University of Milan in collaboration with colleagues from the Department of Materials Science at the Friedrich-Alexander University of Erlangen-Nuremberg in Germany, in a paper recently published in Nature Communications, have shown that through artificial intelligence it is possible to predict the fracture of silica glasses by examining their microstructure.
Thanks to recent advances in deep learning, it is possible to obtain accurate fracture predictions even for highly disordered solids such as glasses. Unfortunately, however,the huge number of parameters used by artificial neural networks often makes a physical interpretation of the results impossible. This problem affects not only fracture prediction but is found in multiple applications of artificial intelligence. Researchers at the Center for Complexity and Biosystems applied a method to identify the areas of the microstructural image most used by the neural network for fracture prediction. This provided insight into what characteristics make a material more susceptible to fracture. "Neural networks are black boxes"-explains Stefano Zapperi, professor of theoretical matter physics and coordinator of the research-"and this is an important limitation in scientific research where the main purpose is to explain the origin of a phenomenon. Thanks to the method we used, it was possible to better understand what are the relevant aspects that determine the fracture of the material and thus obtain not only a prediction but also a greater fundamental understanding of the mechanics of glasses." "The strategy we developed lends itself to further applications"-adds Roberto Guerra, associate professor in the physics department and co-author of the paper-"such as to design disordered materials with better fracture toughness properties."
Read the paper:
Font-Clos, F., Zanchi, M., Hiemer, S. et al. Predicting the failure of two-dimensional silica glasses. Nat Commun 13, 2820 (2022).
https://doi.org/10.1038/s41467-022-30530-1